AI in Environmental Conservation: Innovative Use Cases, Success Stories, and Challenges
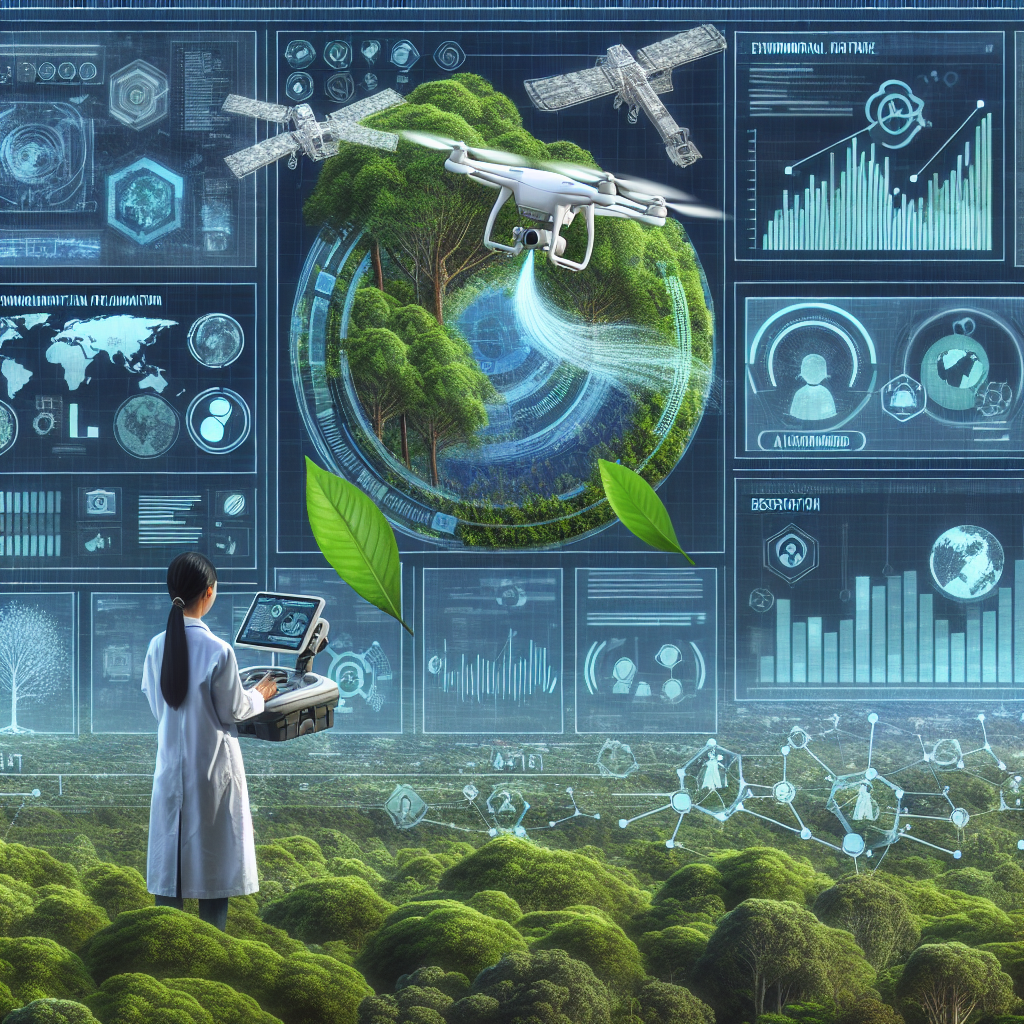
```html
Artificial Intelligence (AI) is driving innovation across various industries, and the realm of environmental conservation is no different. AI has emerged as a powerful tool in tackling some of the most pressing environmental challenges of our time. In this post, we'll explore a range of AI use cases in environmental conservation, share success stories, and reflect on lessons learned from past implementations.
Wildlife Monitoring and Protection
AI-powered technologies are revolutionizing wildlife monitoring and protection efforts. AI systems can analyze data from camera traps, drones, and acoustic sensors to identify and monitor wildlife populations. For instance, Conservation AI Hub uses machine learning to analyze images from camera traps, helping researchers identify species and track their movements.
Despite the benefits, challenges exist. An AI-driven wildlife monitoring project faced issues when the algorithm misclassified species due to lack of diverse training data. This underscores the need for diverse and comprehensive datasets to improve AI accuracy in wildlife identification.
Predicting and Preventing Deforestation
AI is playing a crucial role in the fight against deforestation. By analyzing satellite imagery and forest data, AI algorithms can predict deforestation hotspots and enable early intervention. Global Forest Watch leverages AI to provide real-time data on deforestation events, empowering governments and organizations to take swift action.
However, a project aimed at predicting deforestation faced setbacks when cloud cover and weather conditions affected the accuracy of satellite imagery. This experience highlights the importance of incorporating multiple data sources and developing robust algorithms to handle varying environmental conditions.
Ocean Health Monitoring
AI is enhancing our ability to monitor and protect ocean health. AI-powered tools analyze data from underwater drones, sensors, and satellite imagery to monitor marine ecosystems, track pollution levels, and detect illegal fishing activities. The Ocean Cleanup project uses AI to identify and map plastic debris in the ocean, aiding in large-scale cleanup efforts.
Nevertheless, challenges remain in processing and interpreting the vast amounts of data collected from the oceans. An AI-driven marine monitoring initiative experienced difficulties in accurately identifying pollution sources due to limited data resolution. This highlights the need for advanced data processing techniques and collaboration with marine experts to improve AI accuracy.
Climate Change Modeling and Prediction
AI is instrumental in climate change modeling and prediction. Machine learning algorithms analyze historical climate data, weather patterns, and environmental variables to create predictive models. For example, ClimateAI uses AI to predict climate-related risks and impacts on agricultural production, helping farmers adapt to changing conditions.
However, the complexity of climate systems poses challenges. A climate prediction project encountered issues when AI models failed to account for certain climate anomalies, leading to inaccurate forecasts. This underscores the importance of continuous improvement and validation of AI models to ensure reliable climate predictions.
Renewable Energy Optimization
AI is driving efficiency in the renewable energy sector by optimizing energy production and consumption. AI algorithms analyze data from solar panels, wind turbines, and energy grids to predict energy output, optimize maintenance schedules, and manage energy distribution. Google's DeepMind uses AI to predict wind farm output, increasing the efficiency of renewable energy sources.
Despite the advantages, challenges exist in integrating AI with existing energy systems. A renewable energy project faced difficulties in adapting AI models to regional variations in energy production and demand. This emphasizes the need for adaptable AI solutions that can accommodate diverse environmental and energy contexts.
The Future of AI in Environmental Conservation
The future of AI in environmental conservation holds immense potential. Emerging technologies such as AI-powered robotic systems, advanced remote sensing, and real-time data analytics promise to further enhance conservation efforts. Collaborative initiatives between AI developers, environmental scientists, and policymakers will be crucial in addressing global environmental challenges.
To fully harness AI's potential, it is essential to ensure ethical AI practices, data transparency, and inclusivity. Engaging local communities and stakeholders in AI-driven conservation projects will foster a more holistic and sustainable approach to environmental protection.
In conclusion, AI is transforming environmental conservation through wildlife monitoring, deforestation prevention, ocean health monitoring, climate change modeling, and renewable energy optimization. Success stories from initiatives like Conservation AI Hub and The Ocean Cleanup illustrate AI's potential, while lessons from challenges emphasize the need for diverse data, advanced algorithms, and continuous collaboration. As AI technology advances, its role in preserving our planet will undoubtedly expand, offering new opportunities for sustainable environmental stewardship.
```