Use Cases for AI: Success Stories and Lessons Learned
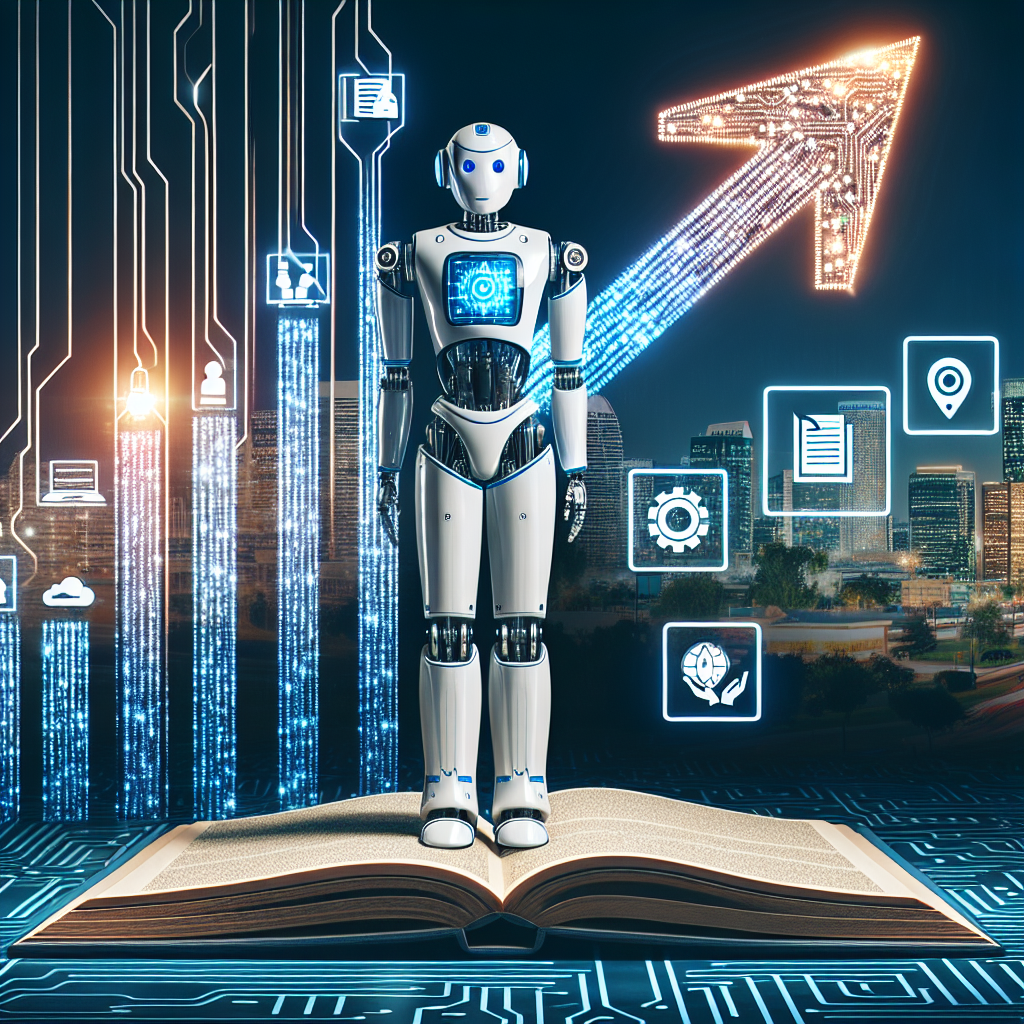
Artificial Intelligence (AI) has become a transformative force across multiple industries, enhancing productivity, efficiency, and even creativity. While the technology is often associated with futuristic and overly technical applications, its real-world impact is evident in various practical use cases that deliver tangible benefits. In this blog post, we'll explore some compelling use cases for AI, showcasing both success stories and lessons learned from failures that offer valuable insights.
Healthcare: Enhancing Diagnostics and Patient Care
The healthcare industry is one of the most promising fields for AI applications. Advanced AI algorithms are now capable of analyzing medical images with remarkable accuracy, assisting doctors in diagnosing conditions like cancer, heart disease, and neurological disorders at their early stages. One notable success story is the implementation of IBM's Watson for Oncology, which aids oncologists by providing evidence-based treatment options derived from vast volumes of medical literature.
However, not all AI ventures in healthcare have been smooth sailing. Google's DeepMind faced criticism when its AI system, designed to predict kidney disease, suffered setbacks due to data privacy concerns and integration challenges with existing healthcare systems. This failure underscores the importance of robust data governance and interoperability in AI healthcare solutions.
Finance: Fraud Detection and Risk Management
AI's ability to process vast amounts of data in real-time has revolutionized the financial sector, particularly in fraud detection and risk management. Companies like MasterCard and Visa employ AI-driven systems that analyze transaction patterns to detect and prevent fraudulent activity. These systems learn continuously, improving their accuracy over time, which greatly reduces the risk of false positives and enhances security.
On the flip side, the financial industry has also seen AI-driven trading algorithms make headlines for the wrong reasons. Flash crashes, triggered by high-frequency trading bots operating on flawed AI models, have led to significant market disruptions. These instances highlight the critical need for rigorous testing and safeguards to ensure AI systems behave predictably in dynamic market conditions.
Retail: Personalization and Inventory Management
Retail giants like Amazon and Walmart have harnessed AI to offer personalized shopping experiences and optimize inventory management. AI algorithms analyze customer behavior, purchase history, and preferences to recommend products, significantly enhancing customer satisfaction and boosting sales. Additionally, AI-powered demand forecasting models help retailers maintain optimal inventory levels, reducing waste and improving logistics.
Despite these successes, some AI implementations in retail have faltered. For instance, attempts at automating pricing strategies using AI have sometimes backfired, leading to pricing errors that frustrated customers and harmed the brand's reputation. This serves as a lesson in the need for ongoing monitoring and human oversight to correct unexpected Ai behaviors promptly.
Manufacturing: Predictive Maintenance and Quality Control
In manufacturing, AI is playing a significant role in predictive maintenance and quality control. AI systems use sensor data from machinery to predict failures before they happen, allowing for timely maintenance that minimizes downtime and reduces costs. Companies like Siemens and General Electric have successfully implemented AI-driven predictive maintenance solutions, achieving substantial efficiency gains and cost savings.
Nevertheless, some manufacturers have encountered challenges with AI adoption. One major lesson is the difficulty in scaling pilot projects to full production environments, where variability and complexity can hinder AI performance. This highlights the necessity for scalable AI models that can adapt to the intricacies of real-world operations.
AI's potential is vast and multifaceted, presenting opportunities to innovate and improve various aspects of society. While success stories demonstrate its transformative power, the lessons learned from failures emphasize the importance of careful planning, ethical considerations, and continuous improvement. As AI continues to evolve, its applications will undoubtedly expand, offering new and exciting possibilities for industries worldwide.